RNNoise: Learning Noise Suppression
Sep. 26th, 2017 10:24 pm![[personal profile]](https://www.dreamwidth.org/img/silk/identity/user.png)
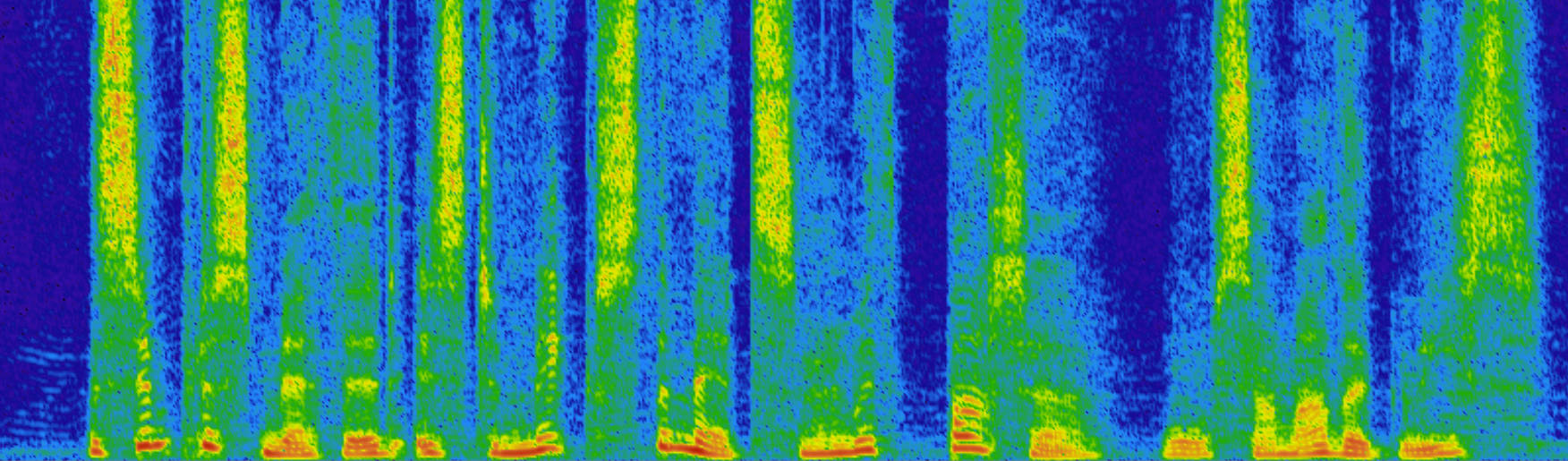
This demo presents the RNNoise project, showing how deep learning can be applied to noise suppression. The main idea is to combine classic signal processing with deep learning to create a real-time noise suppression algorithm that's small and fast. No expensive GPUs required — it runs easily on a Raspberry Pi. The result is much simpler (easier to tune) and sounds better than traditional noise suppression systems (been there!).
Possible alternative uses for this algorithm ?
Date: 2017-09-29 07:49 am (UTC)Your demo got me thinking: if I want to remove something very specific from one track instead of learning a generalized filter, can I train this model with a smaller dataset, like a few seconds from that track?
Re: Possible alternative uses for this algorithm ?
From: (Anonymous) - Date: 2017-09-29 02:07 pm (UTC) - ExpandRe: Possible alternative uses for this algorithm ?
From:Re: Possible alternative uses for this algorithm ?
From:Fab!
Date: 2017-09-29 03:34 pm (UTC)Re: Fab!
From: (Anonymous) - Date: 2017-10-02 12:27 am (UTC) - ExpandRe: Fab!
From: (Anonymous) - Date: 2017-10-02 12:30 am (UTC) - ExpandRe: Fab!
From:Re: Fab!
From: (Anonymous) - Date: 2022-04-23 01:50 pm (UTC) - ExpandWhat about reducing noise in music?
Date: 2017-10-02 03:56 pm (UTC)Re: What about reducing noise in music?
From:Re: What about reducing noise in music?
From: (Anonymous) - Date: 2017-10-06 03:47 pm (UTC) - Expandno subject
Date: 2017-10-03 01:36 pm (UTC)Impressive Work! Really nice results too. I've been working on a similar project but to be exclusively used with Ardour so it's an lv2 plugin (https://github.com/lucianodato/noise-repellent). I used ME method (Rangachari and Loizou) to estimate noise with it but this method seems to work miles better. Do you mind if I make an lv2 plugin out of your library?
Also did you evaluate using discrete wavelet transform instead of fft+bark scale? I've read in the past few works that get near zero latency with that architecture.
Thank you very much for this. It already taught me few things wasn't aware of.
(no subject)
From:ladspa-plugin
From: (Anonymous) - Date: 2017-10-07 11:44 am (UTC) - ExpandAlso did you evaluate using discrete wavelet transform instead of fft+bark scale?
From: (Anonymous) - Date: 2018-01-17 06:49 pm (UTC) - ExpandRESIDUAL NOISE PROBLEM
Date: 2017-10-09 08:21 am (UTC)According to the audio samples you provided, it seems that the RNNoise has more residual noise than the speex. Do you think it will perform better with more noise samples for training?
no subject
Date: 2017-10-30 03:54 am (UTC)Possible Use Cases
Date: 2017-12-03 09:02 pm (UTC)I was wondering if this could be applicable to active noise cancellation as well, with a real time algorithm say from a raspberry pi or maybe a hardware implementation on a fpga. Of course, this would have to be trained on a different training set; I was thinking the Aurora II? Could it be paired with another algorithm such as beamforming to also attenuate noise not from a specific relative location?
Sorry for the plethora of questions.
Thanks.
Re: Possible Use Cases
From:Re: Possible Use Cases
From: (Anonymous) - Date: 2019-01-02 04:43 pm (UTC) - ExpandRe: Possible Use Cases
From:Real-time Algorithm
Date: 2017-12-05 02:48 pm (UTC)But how can we modify to make it real-time spectogram since you purposely delayed it by a few seconds?
Thank you sir.
Using RNNoise as VAD source / Way to improve VAD
Date: 2017-12-08 08:28 am (UTC)First of all, thanks for great work.
Recently, I needed VAD on my application and found that RNNoise has VAD output. So I tried use VAD output of RNNoise.
I commented out code not related with VAD, and made prototype of the app. It works quite nicely despite its small calculation size. But on some audio samples, VAD fails.
I'm trying to improve VAD by adding feature size and neural net size.
Can you give me some hint to improve VAD quality?
Amateur Radio
Date: 2017-12-16 08:08 am (UTC)In Amateur Radio noise suppression is always a big topic. I've added your project into a SDR receiver - if you're not into SDR see www.sdr-radio.com . Anyway it's working well when the audio is above the noise, when the noise and audio are both at the same level I can lose the audio.
This isn't a complaint - just an observation, your code isn't really designed for this situation. Now if only I could get you interested the Amateur Radio your skills would have a big impact on noise reduction.
Thanks for this project, I'll be following it closely.
Re: Amateur Radio
From: (Anonymous) - Date: 2017-12-16 08:09 am (UTC) - ExpandCutting out environmental noise
Date: 2018-01-11 08:47 am (UTC)We have quite poor quality recordings so I thought your algorithm may be quite useful to remove background noise so we can more easily detect voice (which otherwise we cannot at all detect using a webrtcVAD algorithm). I'm just setting it up now. Thought I'd put this here in case you had any idea whether your algorithm would work like I hope.
Thanks a lot,
Re: Cutting out environmental noise
From: (Anonymous) - Date: 2018-06-07 10:37 am (UTC) - ExpandThe Audio Player is awfully nice!
Date: 2018-02-16 04:34 pm (UTC)slightly off topic but how did you do that?
Re: The Audio Player is awfully nice!
From:Training Data
Date: 2018-03-12 02:14 pm (UTC)Spectral non-stationarity metric
Date: 2018-06-20 11:22 am (UTC)Re: Spectral non-stationarity metric
From:Re: Spectral non-stationarity metric
From: (Anonymous) - Date: 2018-08-27 06:39 am (UTC) - ExpandAsking permission to use Javascript version of RNNoise
Date: 2018-08-02 10:18 am (UTC)Re: Asking permission to use Javascript version of RNNoise
From:Re: Asking permission to use Javascript version of RNNoise
From: (Anonymous) - Date: 2018-08-03 03:12 am (UTC) - ExpandInput and output data dimensions
Date: 2018-11-08 01:20 pm (UTC)Many thanks for publishing your exciting work and sharing your code.
I've two points which are not 100% clear to me after reading your documentations and code:
(1) Network training input and output data samples are finite sequences of 42- and 23-element vectors, respectively. But in the operation mode, the trained network is fed sequentially with a single input vector and outputs a single vector?
(2) Is the training data extracted from overlapping spectrogram segments?
Kind regards
Re: Input and output data dimensions
From:Re: Input and output data dimensions
From: (Anonymous) - Date: 2018-11-08 04:33 pm (UTC) - ExpandNegative SNR cases
Date: 2019-01-24 05:44 am (UTC)This application is working very well. But If I give -6dB/-3dB SNR case inputs, some part of speech is corrupted
Processing stereo input seems to work great
Date: 2019-02-28 05:08 pm (UTC)Appears stereo is working fine. However processing just one channel did a slightly better job.
Do you know of any consultants that may be able to assist in our project. want to try to test this inserted realtime on the server and in client of webrtc app as a proof of concept.
Also trying to understand stereo AEC methods.
training
Date: 2019-03-01 05:53 am (UTC)Thanks for this great project. I'd like to train this suppression myself and have downloaded the github version, but I don't see any scripts or way of creating the noisy mixtures as you've described in your paper (with the random filtering, etc.). Are you willing to share the training scripts with us?
Thanks,
Chris
I still have a question about the loss function you define on training this model
Date: 2019-06-27 09:16 am (UTC)I have downloaded your code and got the whole training and evaluation process work.
But reading the code, I find that in the code you generate the data to train the network, you set the label--g[i], to -1 when there is noise only or there is no voice.
And when you define the loss funcion--mycost, you use K.sqrt(y_true) in it.
How can tensorflow or keras do sqrt(-1)? It seems impossible to me.
But it confuses me that I can train the model and get good denoising effect by using your code.
How does that work?????
Filter out a known sound source
Date: 2019-06-30 06:22 am (UTC)I know that you have moved on a bit, but I was wondering about filtering out a known noise source like the output from speakers.
I would like to use this to enhance voice recognition whilst I am listening to internet radio.
So there would be a basis for filtering albeit with the delay and resonance added by the room.
Would this be possible and could you suggest how I might go about it.
Thanks, Greg
Part of speech is corrupted when integrated with webRTC
Date: 2019-10-16 03:02 am (UTC)Re: Part of speech is corrupted when integrated with webRTC
From: (Anonymous) - Date: 2019-10-16 03:22 am (UTC) - ExpandRe: Part of speech is corrupted when integrated with webRTC
From: (Anonymous) - Date: 2020-06-05 08:53 am (UTC) - Expandmemory and mips requirements for RNN
Date: 2019-12-02 01:30 pm (UTC)Do we know the rough memory and mips requirements for running RNN library ?
no subject
Date: 2019-12-25 06:45 pm (UTC)rnnoise demo page down
Date: 2020-05-09 07:09 pm (UTC)