RNNoise: Learning Noise Suppression
Sep. 26th, 2017 10:24 pm![[personal profile]](https://www.dreamwidth.org/img/silk/identity/user.png)
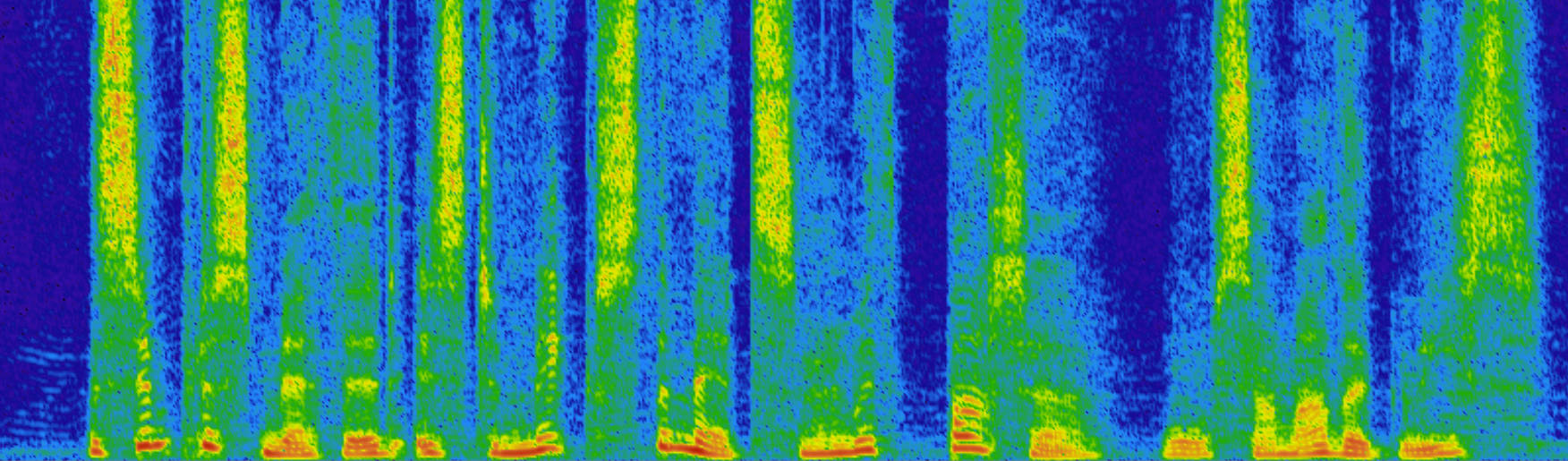
This demo presents the RNNoise project, showing how deep learning can be applied to noise suppression. The main idea is to combine classic signal processing with deep learning to create a real-time noise suppression algorithm that's small and fast. No expensive GPUs required — it runs easily on a Raspberry Pi. The result is much simpler (easier to tune) and sounds better than traditional noise suppression systems (been there!).
Input and output data dimensions
Date: 2018-11-08 01:20 pm (UTC)Many thanks for publishing your exciting work and sharing your code.
I've two points which are not 100% clear to me after reading your documentations and code:
(1) Network training input and output data samples are finite sequences of 42- and 23-element vectors, respectively. But in the operation mode, the trained network is fed sequentially with a single input vector and outputs a single vector?
(2) Is the training data extracted from overlapping spectrogram segments?
Kind regards
Re: Input and output data dimensions
Date: 2018-11-08 02:18 pm (UTC)2) Yes, we use a frame size of 20 ms, with 10 ms overlap.
Re: Input and output data dimensions
Date: 2018-11-08 04:33 pm (UTC)Regarding 2), I think I have to specify my question:
Looking at your training code (rnnoise/training/rnn_train.py), you feed the network with sequences of 2000 42-element vectors/frames (= 1 training sample). Now I wonder if two distinct training samples might share a certain number of frames?